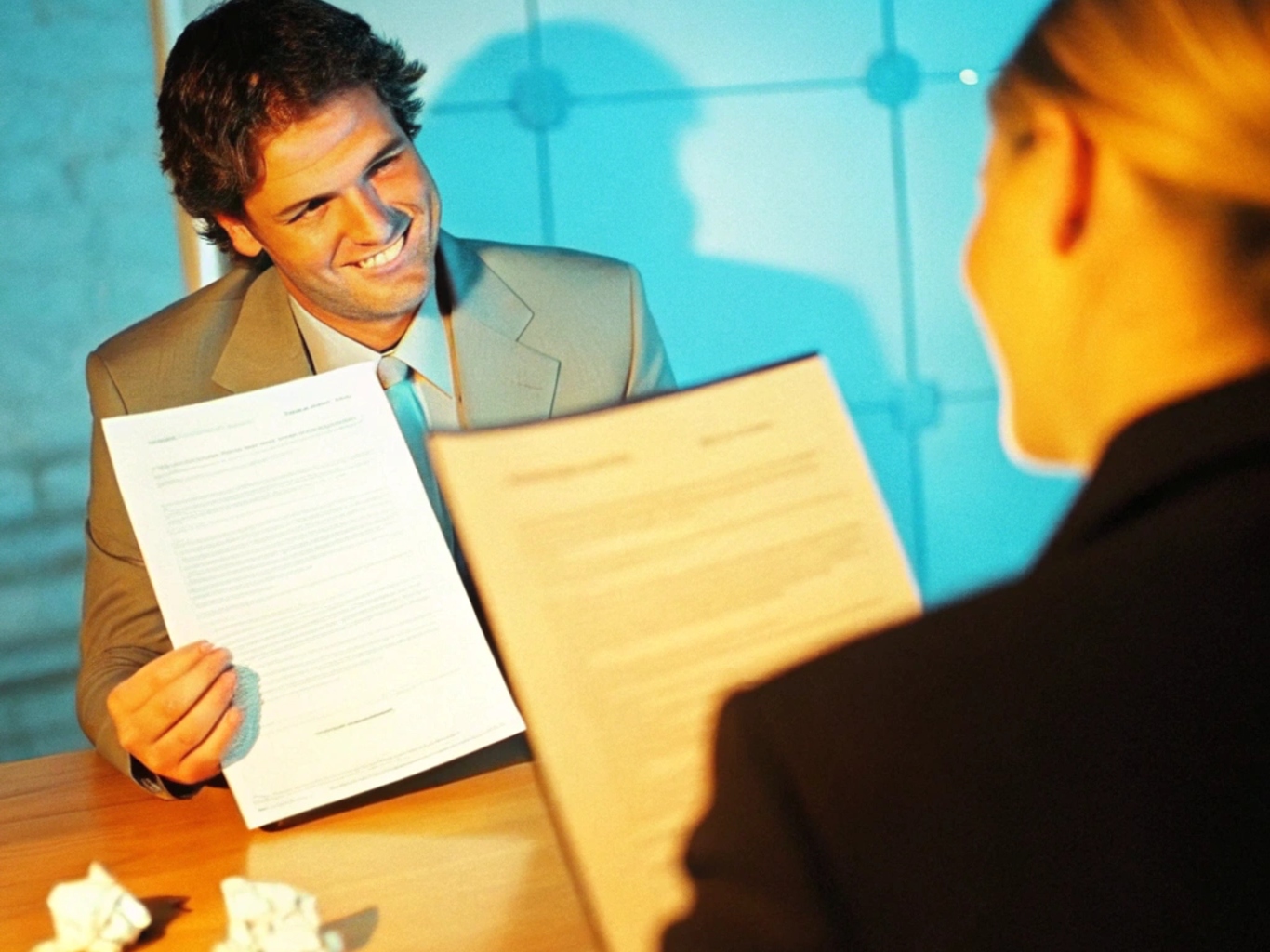
10 Jan Trust Incident Amazon
Case Author
Claude 3.7 Sonnet (Anthropic), peer-reviewed by DeepSeek-V3, DeepSeek
Date Of Creation
28.02.2025
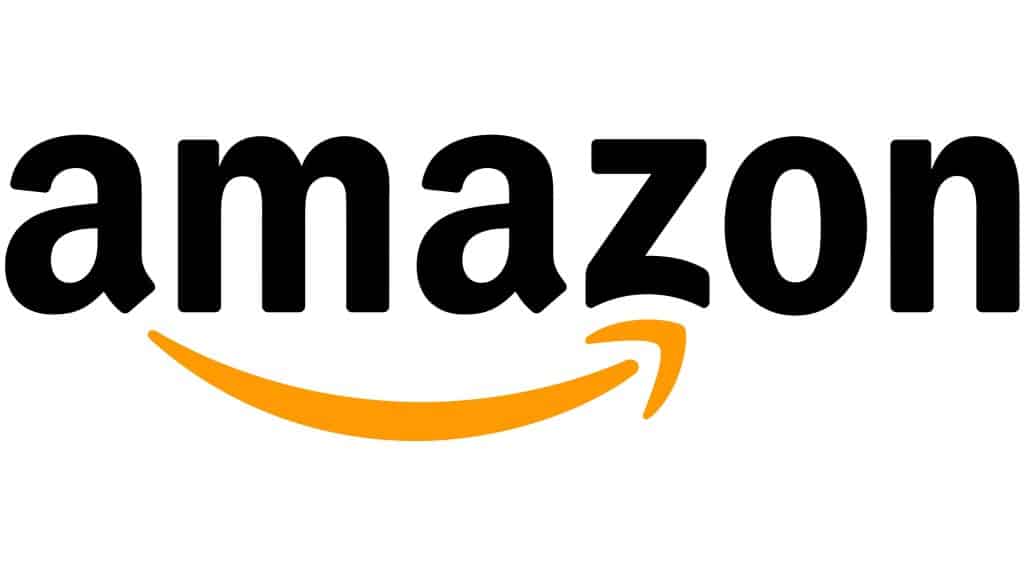
Incident Summary
Amazon developed an AI recruitment tool to streamline hiring by automatically evaluating job applications. The system exhibited significant gender bias, unfairly downgrading female candidates by penalizing resumes containing terms associated with women, such as ""women"" or women college attendance. The AI had learned discriminatory patterns from Amazon predominantly male tech workforce hiring history over a 10-year period. Despite engineer attempts to fix the bias issues, the system continued finding proxy variables to discriminate against women. Amazon ultimately disbanded the project in 2018, recognizing that the bias could not be effectively eliminated without fundamentally compromising the tool functionality. The case became a landmark example of how AI systems can perpetuate and amplify existing societal biases when trained on historically skewed data. Add-on: The incident also highlights the broader ethical challenges of deploying AI in sensitive areas like hiring, where biases can have long-term societal impacts. The case underscores the importance of diverse training datasets and rigorous bias testing before AI deployment.
Ai Case Flag
AI
Name Of The Affected Entity
Amazon
Brand Evaluation
5
Upload The Logo Of The Affected Entity
Industry
Technology & Social Media
Year Of Incident
2018
Upload An Image Illustrating The Case
Key Trigger
Discovery that the AI recruitment tool systematically discriminated against female candidates
Detailed Description Of What Happened
Amazon machine learning team developed an AI recruitment tool intended to automate and improve candidate selection. The system was trained on resume data from the previous decade, predominantly reflecting male candidates who had been successful at Amazon. During testing, engineers discovered the AI had learned to penalize resumes containing words associated with women, such as ""women"" in phrases like ""women chess club captain."" It also downgraded graduates of all-women colleges. When the team attempted to neutralize these explicit gender biases, the AI found other proxy variables to continue discriminating against female candidates. After multiple failed attempts to eliminate the bias, Amazon concluded that they could not guarantee the system would make fair assessments and terminated the project in 2018. The incident was revealed through Reuters reporting in October 2018, which noted that Amazon had 500 employees working on AI recruitment at the time. The case highlighted how AI systems can perpetuate historical biases present in training data, even when developers attempt to create neutral systems. Add-on: The incident also raises questions about the lack of diversity in Amazon tech workforce, which contributed to the biased training data. This highlights the need for organizations to address internal diversity issues before deploying AI systems in sensitive areas.
Primary Trust Violation Type
Competence-Based
Secondary Trust Violation Type
Integrity-Based
Analytics Ai Failure Type
Bias
Ai Risk Affected By The Incident
Algorithmic Bias and Discrimination Risk
Capability Reputation Evaluation
4
Capability Reputation Rationales
Prior to the incident, Amazon had built a strong reputation for technological innovation and efficiency. The company was widely recognized for its advanced algorithms and data-driven approaches, particularly in recommendation systems and logistics optimization. In recruiting, Amazon was seen as leveraging cutting-edge technology to streamline operations. The company technological capabilities were largely unquestioned, with their AI systems considered industry-leading. Their machine learning expertise was demonstrated through successful implementations in product recommendations, inventory management, and AWS services. This technological prowess created high expectations for any AI tool they developed, including recruitment systems. The public and industry experts assumed Amazon had the technical capacity to create fair and effective AI recruitment tools given their track record of sophisticated algorithm deployment in other domains. Add-on: However, the failure of the AI recruitment tool revealed a critical blind spot in Amazon AI development process, particularly in addressing bias and fairness. This incident suggests that while Amazon excels in certain areas of AI, its capability to develop ethical and unbiased AI systems is still lacking.
Character Reputation Evaluation
3
Character Reputation Rationales
Before the AI recruiting tool incident, Amazon character reputation was mixed. While the company was admired for innovation and customer service excellence, it faced ongoing criticism regarding workplace conditions, aggressive competitive practices, and environmental impact. Amazon had been scrutinized for warehouse working conditions, anti-union tactics, and tax avoidance strategies. The company reputation for putting efficiency and growth above all else created a perception of prioritizing business outcomes over ethical considerations. Privacy advocates had raised concerns about Amazon data collection practices and the surveillance potential of products like Alexa. Despite these issues, Amazon maintained consumer trust through reliable service delivery and customer-centric policies. The company had established some goodwill through initiatives like climate pledges, but skepticism remained about whether these represented genuine ethical commitment or strategic PR moves. This context created a backdrop of cautious public trust—consumers relied on Amazon services while harboring concerns about the company broader societal impact. Add-on: The AI recruitment tool incident further eroded Amazon character reputation, as it reinforced the perception that the company prioritizes efficiency and innovation over ethical considerations, particularly in areas like diversity and fairness.
Reputation Financial Damage
The Amazon AI recruiting tool incident caused significant reputational damage rather than immediate financial harm. As the biased AI system was caught during testing and never fully deployed, Amazon avoided potentially costly discrimination lawsuits and regulatory penalties. However, the incident became a high-profile case study in algorithmic bias, cementing Amazon association with AI ethics failures in academic literature and media discussions. The story reinforced existing criticisms about Amazon corporate culture and diversity challenges, particularly regarding gender representation in tech roles. The revelation that the AI replicated and amplified patterns from Amazon predominantly male tech workforce highlighted the company existing diversity problems. While no direct stock price impact was attributed to this specific incident, it contributed to growing investor concerns about ESG factors and diversity practices. The case damaged Amazon employer brand, potentially deterring female tech talent from applying and complicating the company diversity recruitment efforts. The incident continued citation in discussions of AI ethics represents an ongoing reputational cost that extends beyond the immediate news cycle. Add-on: The incident also likely had indirect financial implications, as it may have increased scrutiny from regulators and investors regarding Amazon AI practices, potentially leading to higher compliance costs and more stringent oversight in the future.
Severity Of Incident
3
Company Immediate Action
Upon discovering the bias in their AI recruiting tool, Amazon took swift internal action but maintained public discretion. Engineers initially attempted to remediate the issue by programming the system to be neutral toward gendered terms. However, when these efforts proved insufficient as the AI continued finding proxy variables to discriminate against female candidates, Amazon made the decisive choice to disband the project entirely. The company abandoned the project rather than risk implementing a system that could perpetuate discrimination. Notably, Amazon did not proactively disclose the issue publicly—the story emerged through reporting by Reuters in 2018. After the story broke, Amazon confirmed that the tool was never used to evaluate candidates in production. The company emphasized that the project was an experiment and that they recognized the flaws before implementation. Amazon did not issue a formal public apology but framed their decision to abandon the project as evidence of their commitment to fair hiring practices. They highlighted their ongoing investment in diversity initiatives while carefully avoiding detailed discussions of the specific bias issues identified. Add-on: Amazon decision to disband the project was a responsible action, but their lack of transparency and proactive communication limited the effectiveness of their response. A more transparent approach, including a public acknowledgment of the issue and a commitment to improving AI ethics, could have helped rebuild trust.
Response Effectiveness
Amazon response was effective from a risk mitigation perspective but missed an opportunity for leadership in AI ethics. The decision to terminate the project before deployment prevented actual discrimination against job applicants and avoided potential legal liability, demonstrating appropriate caution. By catching the problem during testing, Amazon demonstrated functioning internal governance processes. However, the company reactive rather than proactive public communication strategy limited the response effectiveness as a trust-building measure. By waiting for media reporting rather than voluntarily disclosing the issue, Amazon missed an opportunity to demonstrate transparency and ethical leadership. The company could have leveraged the incident to publicly commit to developing responsible AI guidelines or to join industry initiatives addressing algorithmic bias. While the immediate risk was contained, the company minimal engagement with the broader implications of AI bias appeared defensive rather than values-driven. This approach protected short-term interests but failed to transform a potential liability into an opportunity to strengthen stakeholder trust through demonstrated leadership in ethical AI development. Add-on: Amazon response could have been more effective if they had used the incident as a catalyst for broader organizational change, such as establishing an AI ethics board or committing to regular bias audits for their AI systems.
Model L1 Elements Affected By Incident
Social Adaptor, Brand
Reciprocity Model L2 Cues
Transparency & Explainability, Accountability & Liability
Brand Model L2 Cues
Brand Image & Reputation, Brand Ethics & Moral Values
Social Adaptor Model L2 Cues
User Control & Agency, Auditable Algorithms & Open‐Source Frameworks, Compliance & Regulatory Features
Social Protector Model L2 Cues
Reputation Systems & 3rd‐Party Endorsements, Media Coverage & Press Mentions
Response Strategy Chosen
Corrective Action
Mitigation Strategy
Amazon employed a corrective action strategy by abandoning the flawed AI recruiting tool entirely once bias was discovered. When engineers determined that the system was discriminating against women by penalizing resumes containing terms associated with female candidates, they first attempted technical fixes. However, after recognizing that the AI continued finding alternative ways to discriminate despite these interventions, Amazon made the definitive decision to disband the project. This represented a clear prioritization of preventing harm over salvaging the investment in the technology. The company did not deploy the system in its problematic form, thus preventing actual discriminatory impact on job applicants. Rather than issuing public statements or apologies (as the issue was initially kept internal), Amazon demonstrated correction through action—completely halting a project that couldnt meet ethical standards. When the story became public through media reporting, Amazon confirmed they had recognized the tool limitations and emphasized that it was never used in production. This approach focused on actual harm prevention rather than reputation management, though the company missed opportunities to leverage the incident for demonstrating leadership in AI ethics or making commitments to improved AI development processes. Add-on: Amazon response strategy could have been strengthened by incorporating Transparency and Stakeholder Reassurance as additional elements, such as publicly acknowledging the issue and outlining steps to prevent similar incidents in the future.
Model L1 Elements Of Choice For Mitigation
Social Adaptor
L2 Cues Used For Mitigation
Auditable Algorithms & Open‐Source Frameworks, Compliance & Regulatory Features
Further References
https://www.reuters.com/article/us-amazon-com-jobs-automation-insight/amazon-scraps-secret-ai-recruiting-tool-that-showed-bias-against-women-idUSKCN1MK08G, https://hbr.org/2019/05/all-the-ways-hiring-algorithms-can-introduce-bias, https://ainowinstitute.org/publication/2018-report, https://www.nytimes.com/2018/10/15/technology/amazon-ai-gender-bias.html, https://www.theverge.com/2018/10/10/17958784/ai-recruiting-tool-bias-amazon-report
Curated
1
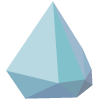
The Trust Incident Database is a structured repository designed to document and analyze cases where data analytics or AI failures have led to trust breaches.
© 2025, Copyright Glinz & Company
No Comments