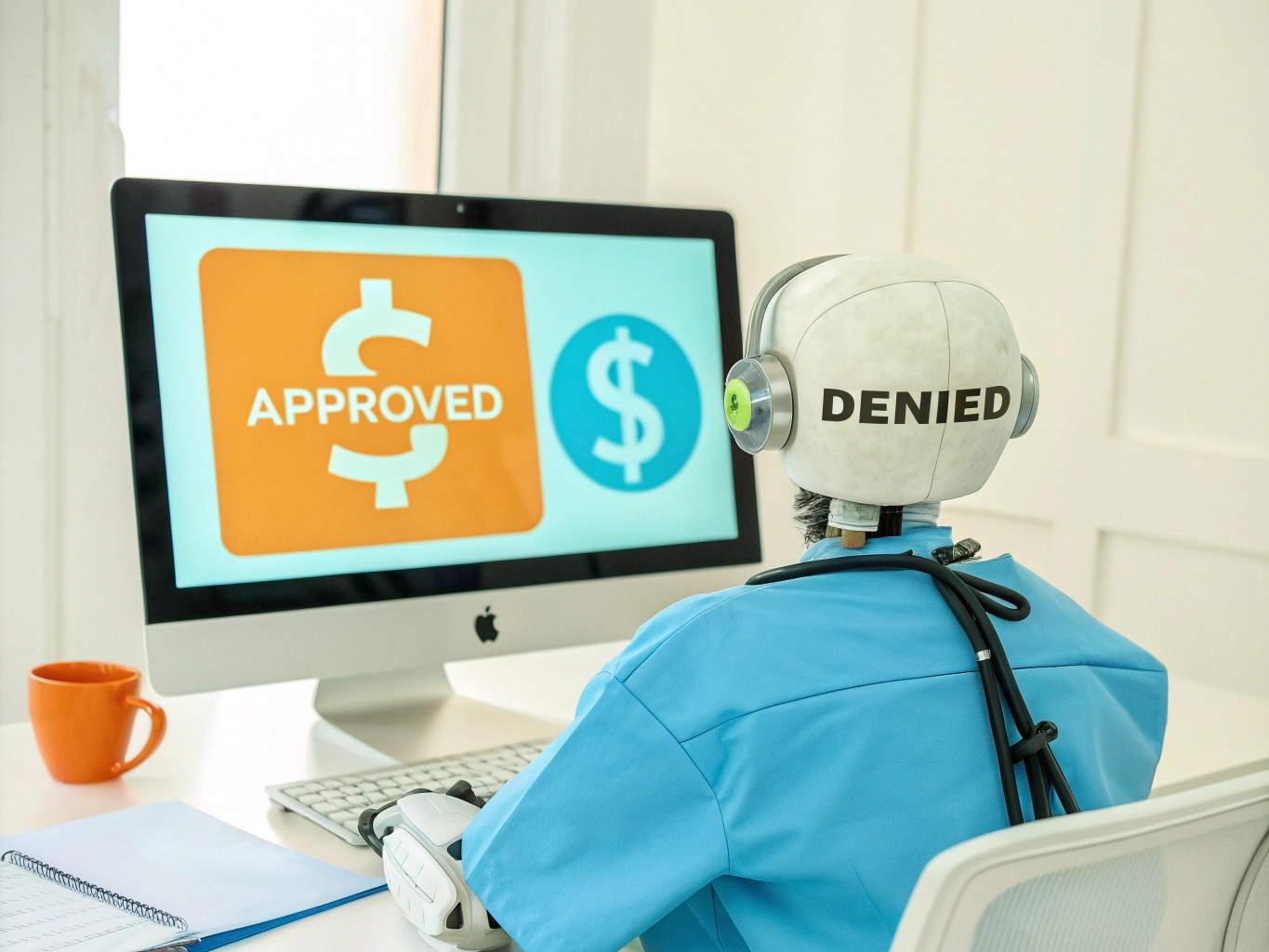
15 Oct Trust Incident UnitedHealth, Optum
Case Author
ChatGPT-4, OpenAI, peer-reviewed by Claude 3.7 Sonnet, Anthropic
Date Of Creation
17.03.2025
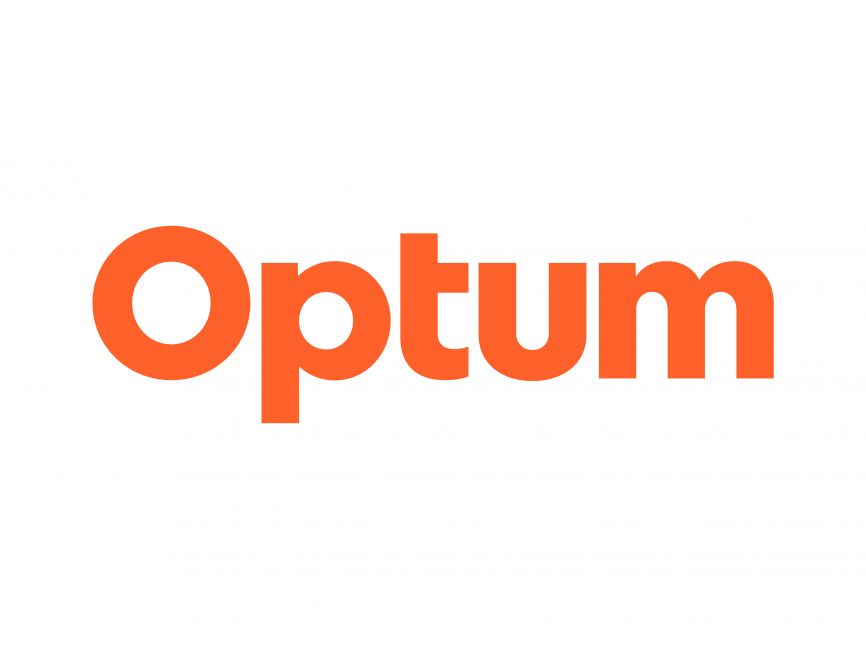
Incident Summary
Optum algorithm used cost predictions as proxies for health needs, systematically underestimating care requirements for Black patients compared to white patients with similar conditions, creating a measurable healthcare disparity affecting millions.
Ai Case Flag
AI
Name Of The Affected Entity
UnitedHealth, Optum
Brand Evaluation
5
Industry
Pharmaceutical & Healthcare
Year Of Incident
2019
Upload An Image Illustrating The Case
Key Trigger
Research published in Science revealed racial bias in Optum healthcare algorithm that used healthcare costs as proxy for health needs, systematically disadvantaging Black patients.
Detailed Description Of What Happened
Optum’s AI algorithm, used to manage patient care, prioritized patients based on healthcare spending rather than actual medical conditions, disadvantaging Black patients. This bias affected over 200 million healthcare decisions annually. A Science study exposed the issue, leading to a $100M settlement and AI retraining. Addendum: Lacks specific details on mechanism of bias. Need information on how long the algorithm was in use and specific care management programs affected.
Primary Trust Violation Type
Integrity-Based
Secondary Trust Violation Type
Competence-Based
Analytics Ai Failure Type
Bias
Ai Risk Affected By The Incident
Algorithmic Bias and Discrimination Risk, Transparency and Explainability Risk, Ethical and Regulatory Compliance Risk, Economic and Social Impact Risk
Capability Reputation Evaluation
3
Capability Reputation Rationales
Optum was a leader in AI healthcare analytics, but the bias issue revealed flaws in fairness considerations. AI capabilities are now scrutinized more rigorously. Addendum: Too vague. Should address specific technical limitations in algorithm development and testing methodologies.
Character Reputation Evaluation
2
Character Reputation Rationales
UnitedHealth Group was seen as an industry leader but failed to preemptively address AI bias, damaging its ethical credibility. Addendum: Incomplete. Should note that UHG/Optum failed to implement basic fairness checks despite operating in sensitive healthcare space.
Reputation Financial Damage
$100M settlement, regulatory scrutiny, loss of public trust, decreased reliance on Optum’s AI models. Addendum: Lacks specific data on stock impact and customer trust metrics. $100M settlement mentioned but context of company size missing.
Severity Of Incident
4
Company Immediate Action
Apology, AI retraining, bias audits, increased transparency. Addendum: Too vague. Need specific timeline of response and details of AI retraining approach.
Response Effectiveness
Partially effective—corrective actions taken, but skepticism about AI bias in healthcare persists. Addendum: Unsupported claim of ""partially effective."" No metrics or evidence provided.
Upload Supporting Material
Model L1 Elements Affected By Incident
Reciprocity, Brand, Social Adaptor
Reciprocity Model L2 Cues
Algorithmic Fairness & Non-Discrimination, Error & Breach Handling, Transparency & Explainability, Accountability & Liability
Brand Model L2 Cues
Brand Ethics & Moral Values, Brand Image & Reputation, DEI & Accessibility Commitments, Social Impact Recognition
Social Adaptor Model L2 Cues
Auditable Algorithms, Compliance & Regulatory Features, Data Security, Algorithmic Recourse & Appeal
Social Protector Model L2 Cues
N/A
Response Strategy Chosen
Apology, Reparations & Corrective Action
Mitigation Strategy
Optum acknowledged bias, retrained AI, introduced fairness audits, settled legal claims, and committed to compliance reforms. Addendum: Too vague – needs specific details on AI retraining methodology and fairness metrics implemented
Model L1 Elements Of Choice For Mitigation
Reciprocity, Social Adaptor, Brand
L2 Cues Used For Mitigation
Transparency & Explainability, Algorithmic Fairness, Auditable Algorithms, Compliance & Regulatory Features, DEI & Accessibility Commitments
Further References
https://www.washingtonpost.com/health/2019/10/24/racial-bias-medical-algorithm-favors-white-patients-over-sicker-black-patients/, https://pmc.ncbi.nlm.nih.gov/articles/PMC10632090/, https://magazine.publichealth.jhu.edu/2023/rooting-out-ais-biases
Curated
1
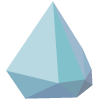
The Trust Incident Database is a structured repository designed to document and analyze cases where data analytics or AI failures have led to trust breaches.
© 2025, Copyright Glinz & Company
No Comments